This study proposes a novel method to automatically generate a large number of handwritten character images from a small dataset, aiming to enhance the training data for a Japanese handwritten character recognition model.
Key features of the proposed method:
- Generation of diverse handwritten character images using style transformation based on Adaptive Instance Normalization (AdaIN)
- Integration of features from the CLIP (Contrastive Language-Image Pre-training) text encoder to reinforce character structural information
This enables the generation of various handwritten character images, including hiragana, katakana, and kanji.
Examples of generated images:
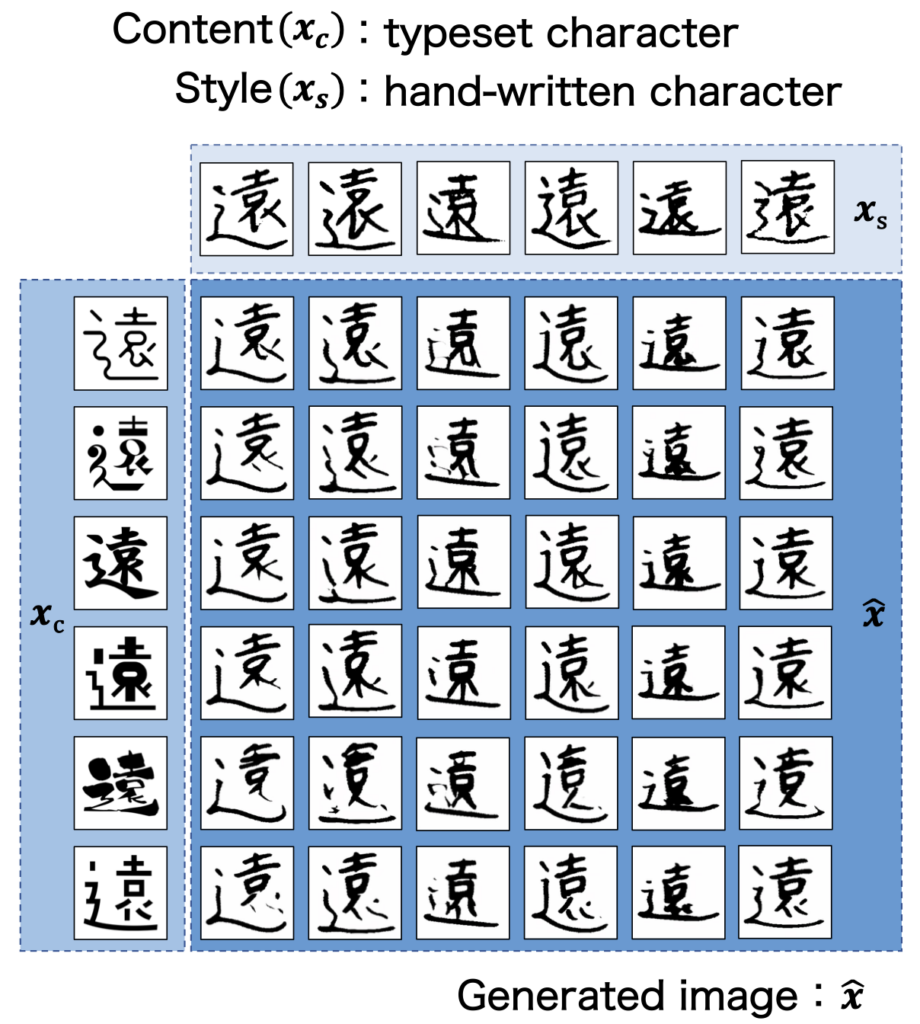
By incorporating the generated handwritten character images into the training data from the ETL database, the accuracy (Macro F1 score) of the character recognition model improved from 0.9733 to 0.9861.
The proposed method is expected to significantly enhance the performance of character recognition models while reducing the cost of collecting handwritten character data.
本研究では、日本語の手書き文字認識モデルの学習データを拡張するため、少量のデータセットから大量の手書き文字画像を自動生成する新手法を提案しています。
提案手法の特徴は以下の2点です。
- Adaptive Instance Normalization (AdaIN)を用いたスタイル変換により、多様な手書き文字画像を生成
- CLIP (Contrastive Language-Image Pre-training)のテキストエンコーダから得た特徴量を統合し、文字の構造情報を強化
これにより、ひらがな・カタカナ・漢字を含む様々な手書き文字画像を生成することができます。生成画像の例を上図に示します。
ETLデータベースの手書き文字画像を学習データに加えることで、文字認識モデルの精度(Macro F1スコア)が0.9733から0.9861に向上しました。
本手法により、手書き文字データの収集コストを削減しつつ、文字認識モデルの性能を大幅に改善できると期待されます。